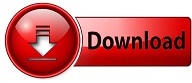
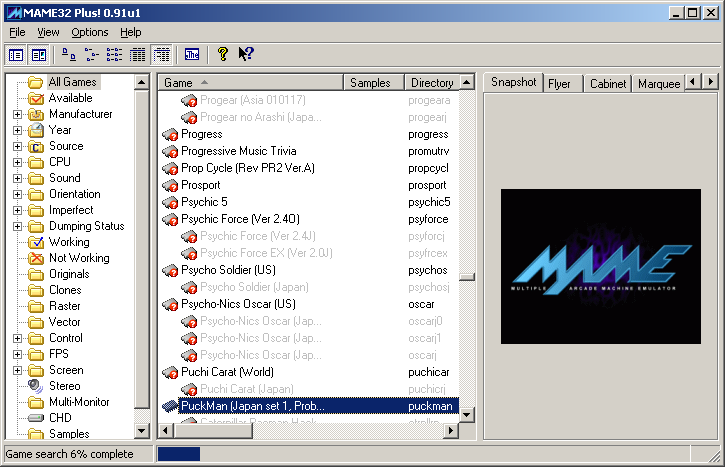

This is, at least partly, due to the heterogeneous methodological quality in radiomic research, caused by the complexity of the analysis pipelines. Despite the growing interest and rising number of research articles being published, radiomics is still far from fulfilling its promise of guiding oncologic imaging toward personalized medicine. Using a multi-step process, radiomics extracts potential biomarkers from medical images that could power decision support tools. Recently, it has been suggested that its importance could be further increased by accessing a new layer of previously hidden quantitative data at the pixel level. Imaging plays a crucial role in the management of oncologic patients, from the initial diagnosis to staging and treatment response monitoring. These findings suggest that the evaluation of the annotators may help tackle the fundamental budget issues in the histopathology domain Furthermore, models trained from data annotated with lower inter-labeler variability outperform those from higher inter-labeler variability. Instead, decreasing the inter-rater variability with the expense of decreasing dataset size increased the model performance. We found that naively increasing the data size at the expense of inter-rater variability does not necessarily lead to better-performing models in cell detection. We propose a method to measure such variability, and by excluding those annotators with low variability, we verify the trade-off between the amount of data and its quality. We present a large-scale study on the variability of cell annotations among 120 board-certified pathologists and how it affects the performance of a deep learning model. Under this setting, the relation between annotators' variability and model performance has received little attention. In particular, annotating different types of cells in histopathology suffer from high inter- and intra-rater variability due to the ambiguity of the task. However, annotating medical images is challenging as it requires expertise and a large budget. Large annotated datasets have been a key component in the success of deep learning.
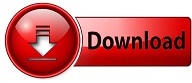